Title: Introducing DETR Document Table Detection: Revolutionizing Table Detection in Images and Documents
Tables are an essential element in documents, playing a crucial role in presenting structured data. With the rapid digitization of information, accurately detecting tables within images and documents has become increasingly important. To address this challenge, a groundbreaking AI model called DETR Document Table Detection has emerged, bringing significant advancements in table detection technology. In this blog post, we will explore the capabilities of DETR Document Table Detection and how it revolutionizes the way we identify both bordered and borderless tables in various contexts.
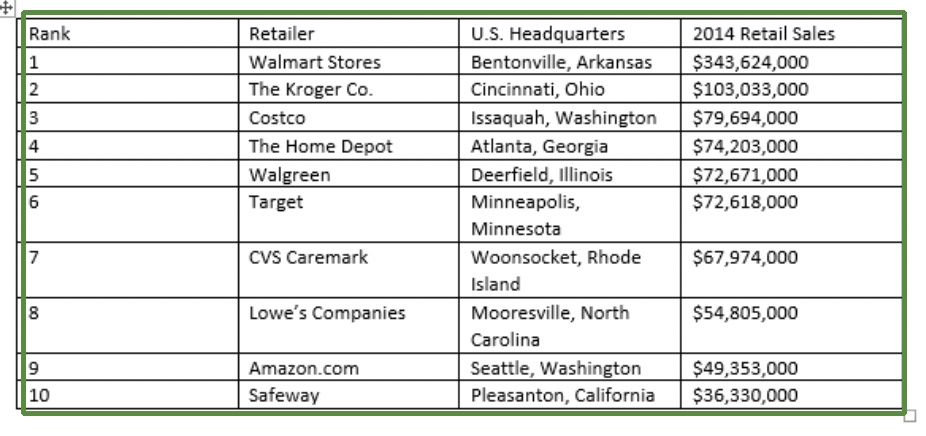
Understanding Table Detection
Table detection is the process of locating tables within documents or images containing textual and tabular data. It serves as a fundamental step in automating data extraction and analysis from scanned papers, research articles, financial reports, and many other sources. Traditional methods for table detection heavily relied on handcrafted features and complex rule-based algorithms, often leading to inconsistent and inaccurate results.
Enter DETR Document Table Detection
DETR Document Table Detection, short for "Detection Transformer for Document Table Detection," represents a significant leap forward in the field of computer vision and document analysis. Developed on the foundation of transformer neural networks, DETR Document Table Detection is a state-of-the-art deep learning model that leverages the power of attention mechanisms to process complex visual patterns.
Key Features and Advantages
- Seamless Detection of Tables in Images and Documents: DETR Document Table Detection is not limited to specific document formats or table styles. It can handle a wide range of image and document types, making it a versatile solution for table detection across different domains.
- Bordered and Borderless Table Detection: Unlike traditional methods that struggled to detect borderless tables accurately, DETR Document Table Detection excels in precisely identifying both bordered and borderless tables. This capability is especially valuable as borderless tables have gained popularity due to their modern and sleek appearance.
- End-to-End Training: DETR Document Table Detection learns to detect tables in an end-to-end manner, eliminating the need for manual feature engineering. This autonomous learning process results in higher accuracy and adaptability to varying table layouts.
- Attention Mechanism: The attention mechanism in DETR Document Table Detection allows the model to focus on relevant parts of the document, enabling it to identify complex tables in cluttered backgrounds effectively.
- Scalability: The model is designed to handle large-scale tasks efficiently, ensuring fast and accurate table detection even in extensive documents or image collections.
- How DETR Document Table Detection Works
- Input Encoding: The document or image containing potential tables is initially encoded into a set of feature vectors, capturing essential visual elements.
- Table Detection Heads: The model then employs table detection heads that specialize in identifying the presence and location of tables within the encoded data.
- Bounding Box Prediction: DETR Document Table Detection predicts the bounding boxes that enclose the detected tables, providing precise spatial information.
- Non-Maximum Suppression: To avoid redundant detections, the model utilizes non-maximum suppression techniques, ensuring each table is identified only once.
Use Cases and Impact
The applications of DETR Document Table Detection are diverse and far-reaching:
- Automated Data Extraction: DETR Document Table Detection serves as a critical component in automating data extraction processes, significantly reducing human effort and potential errors.
- Document Digitization: For historical documents or books, DETR Document Table Detection plays a pivotal role in digitizing tables, preserving valuable information, and facilitating accessibility.
- Financial Analysis: Financial institutions can benefit from automated table detection for efficient analysis of financial reports, leading to more informed decision-making.
- Academic Research: Researchers can leverage DETR Document Table Detection to streamline data extraction from scholarly articles, expediting the literature review process.
Conclusion
DETR Document Table Detection represents a major breakthrough in the domain of table detection, offering accurate and efficient identification of bordered and borderless tables in various documents and images. Its attention-based approach and end-to-end training bring unparalleled advantages, making it a go-to solution for automating data extraction, document digitization, and information analysis. As AI continues to advance, DETR Document Table Detection showcases the immense potential of deep learning models in revolutionizing how we interact with and extract valuable insights from structured data in the digital age.